
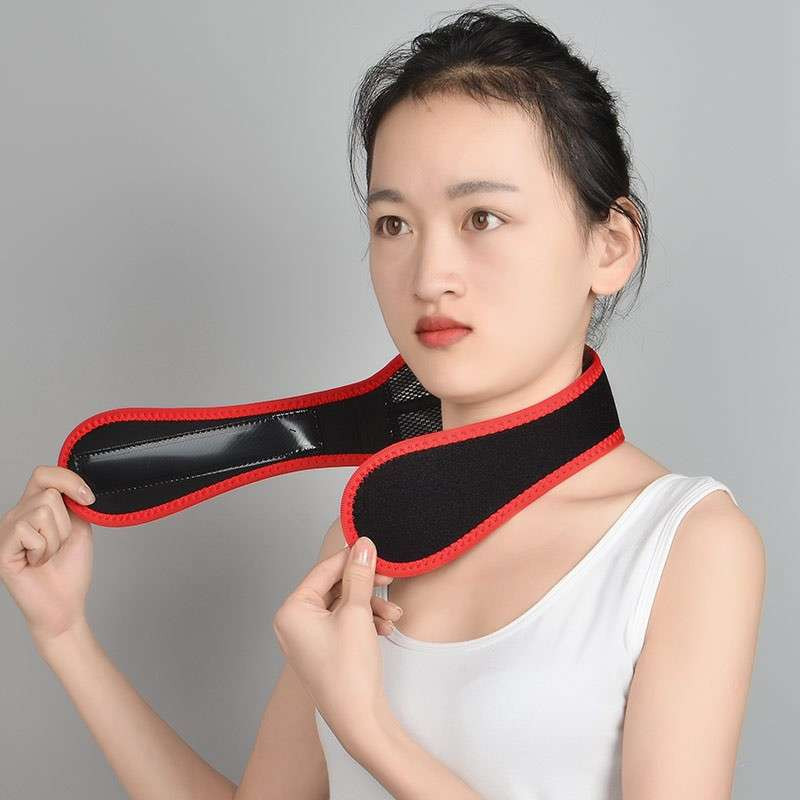
The data has high variance along these dimensions.In general, we are interested in representing the data using fewer dimensions such that, Requirements for Dimensionality Reduction The formula for the correlation coefficient is defined as: We can normalize the correlation to get the correlation coefficient. If two columns are highly correlated (or have high covariance), one is redundant since it is linearly dependent on the other column. Correlationĭata correlation is how one set of data may correspond to another set. In general, we are interested in representing the data using fewer dimensions such that the data has higher variance along these dimensions. In this way, we have reduced the dimensionality. Why not care about $u_2$.īecause the variance in the data in this direction is minimal (all data points have almost the same value in the $u_2$ direction), if we were to build a classifier on top of this data, then $u_2$ would not contribute to the classifier as the points are not distinguishable along this direction. It seems that the same data that was initially in $R_2:(x,y)$ can now be represented in $R_1:(u_1)$ by making an intelligent choice of basis. We can observe that all the points have a minimal component in the direction of $u_2$(almost noise). Here I have used $u_1$ and $u_2$ as a basis instead of $x$ and $y$. Now, what if we choose a different basis? To explain the concept of dimensionality reduction, I will take an example, consider the following data where each point (vector) is represented using a linear combination of the $x$ and $y$ axes: More input features often make a predictive modeling task more challenging to model, generally referred to as the curse of dimensionality. Introduction What is Dimensionality Reduction?ĭimensionality: The number of input variables or features for a dataset is referred to as its dimensionality.ĭimensionality reduction is the transformation of data from a high-dimensional space into a low-dimensional space so that the low-dimensional representation retains some meaningful properties of the original data, ideally close to its intrinsic dimension (number of variables needed in a minimal representation of the data).ĭimensionality reduction refers to techniques that reduce the number of input variables in a dataset. Make sure you understand these terms before going through this blog. Also, readers should be familiar with few terms of linear algebra, like basis vectors, vector spaces, orthogonality, and covariance. The reader should have basic knowledge of linear algebra (matrix operations and their properties) and statistics. Reconstructing images using less information.
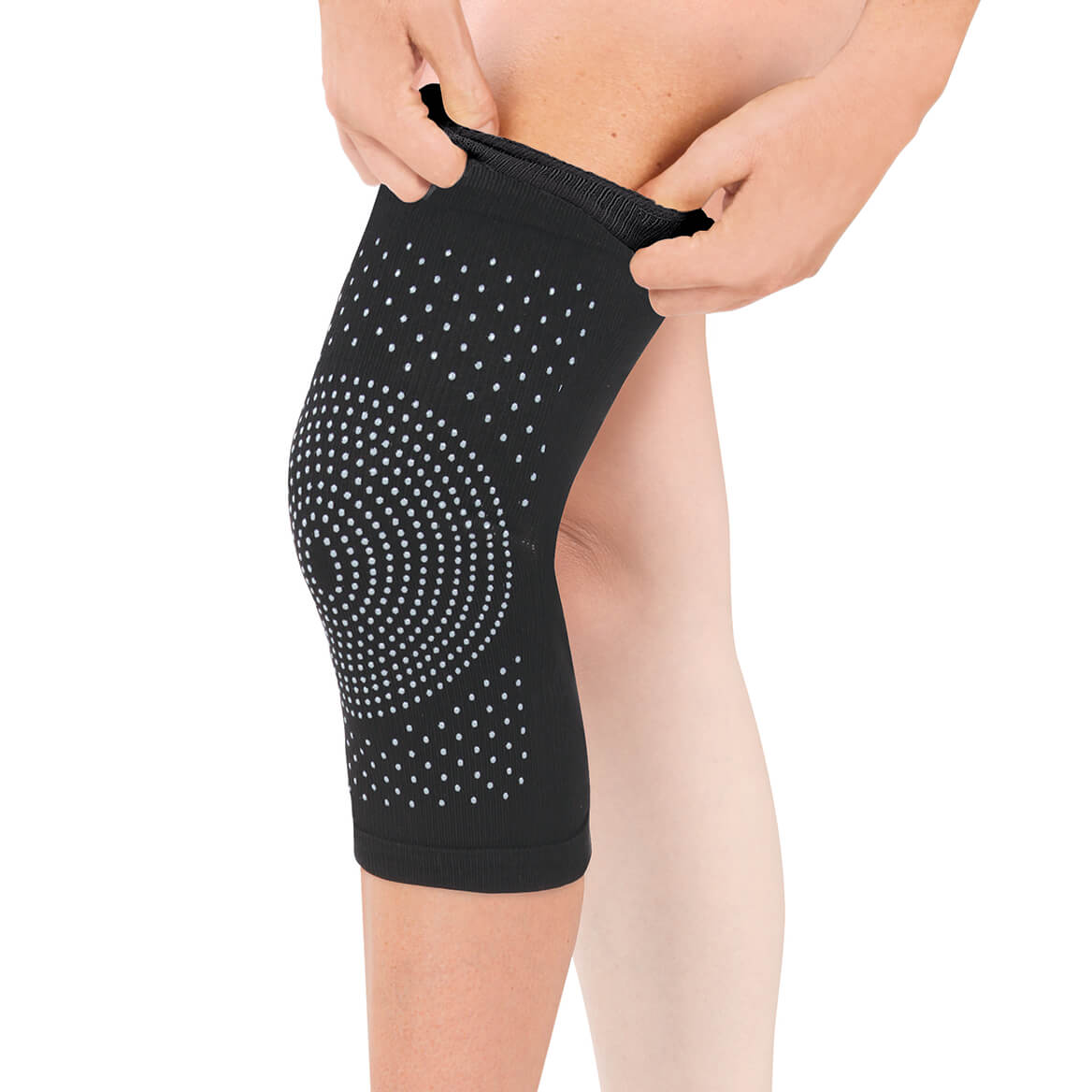
#How to compress picture with infra how to
RELATED: How to Reduce the Size of a Microsoft Word Document If you uncheck this option, PowerPoint will compress all of the images in the presentation, which overrides any changes you may have made to those images. In the “Compression Options” group, you can choose if the compression applies only to the selected picture.
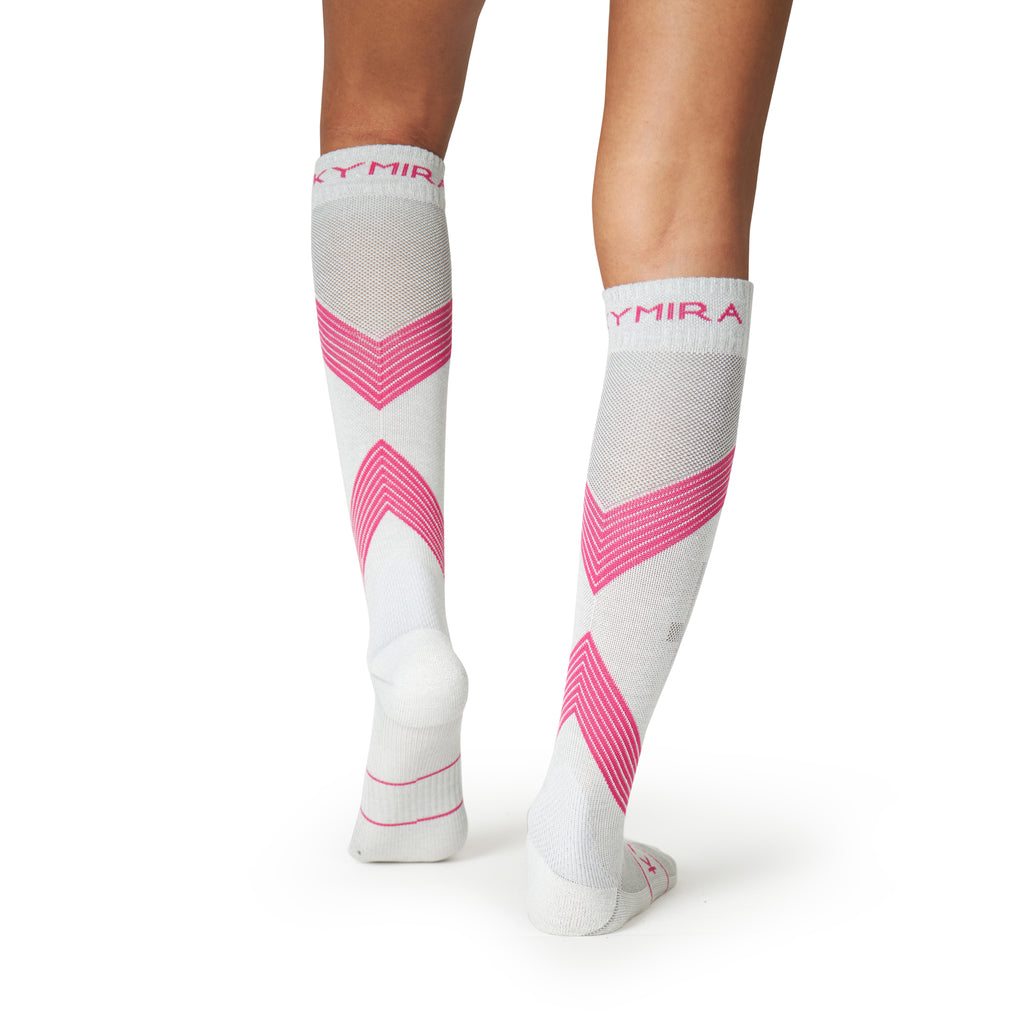
Here, click the “Compress Pictures” button in the “Adjust” group. Once selected, you’ll automatically be in the “Picture Format” tab.

#How to compress picture with infra windows
Compress Images in PowerPoint for Windows
